Between-School Variation in Students’ Achievement, Motivation, Affect, and Learning Strategies: Results from 81 Countries for Planning Cluster-Randomized Trials in Education
Martin Brunner, Uli Keller, Marina Wenger, Antoine Fischbach & Oliver Lüdtke
Does an educational intervention work?
When planning an evaluation, researchers should ensure that it has enough statistical power to detect the expected intervention effect. The minimally detectable effect size, or MDES, is the smallest true effect size a study is well positioned to detect. If the MDES is too large, researchers may erroneously conclude that their intervention does not work even when it does. If the MDES is too small, that is not a problem per se, but it may mean increased cost to conduct the study. The sample size, along with several other factors, known as design parameters, go into calculating the MDES. Researchers must estimate these design parameters. This paper provides an empirical bases for estimating design parameters in 81 countries across various outcomes.
Does an educational intervention work on a larger scale?
Cluster-randomized trials are important for the development of evidence-based educational policies. They allow causal inferences about whether educational interventions work on a large scale. In such studies, entire classes or schools are typically randomized to intervention conditions.
What design parameters are needed to plan cluster-randomized intervention studies at schools?
Previous research has provided design parameters for cluster-randomized trials drawing on U.S. samples for achievement as the outcome. Capitalizing on representative data of 15-year old students, this paper and the online supplementary materials provide design parameters for 81 countries in three broad outcome categories (achievement, affect and motivation, and learning strategies) for domain-general and domain-specific (mathematics, reading, and science) outcome measures. Specifically, we present design parameters to plan cluster-randomized studies where intervention conditions are assigned to schools: information about between-school differences in outcomes (i.e., the intraclass correlation ICC) as well as the amount of outcome variance (i.e., R2) that can be explained by covariates at the student (L1) and school (L2) levels. We used sociodemographic characteristics (i.e., gender, immigration background, and a composite index of students’ socioeconomic background) as covariates.
Strive for an optimal fit between the target research context and the context in which design parameters were obtained!
Between-school differences and the amount of variance explained at L1 and L2 varied widely across countries and outcomes (see Figure 1), demonstrating the need to strive for an optimal fit between the target research context and the context in which design parameters were obtained. We illustrate the use of the design parameters to plan cluster-randomized studies under several scenarios (e.g., how to determine the sample size or how to examine the MDES).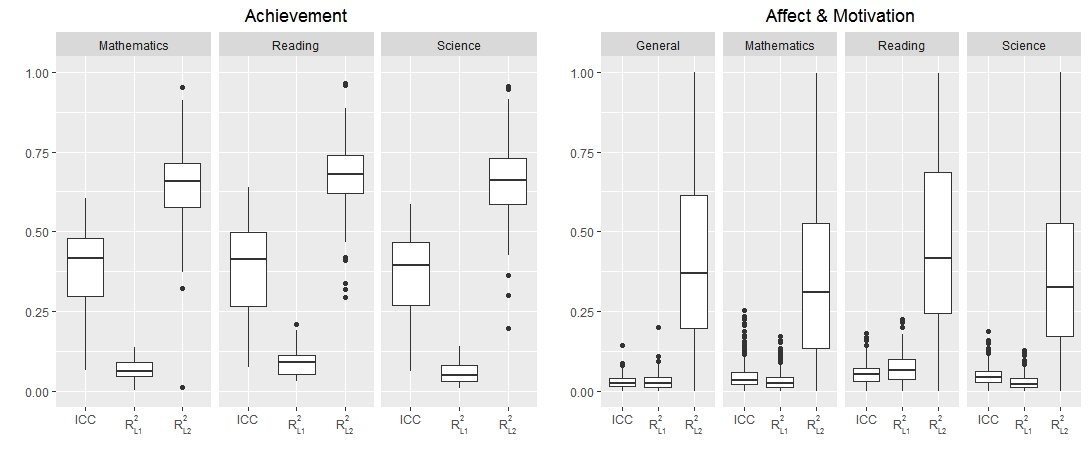
Figure 1. Distribution of Design Parameters Across Countries by Outcome Categories and Domains. The boxplots show the distributions of the intraclass correlations (ICC) and variance explained by sociodemographic characteristics at the individual student (R2L1) and school level (R2L2). Results for the outcome category “learning strategies” (not shown in this figure) were similar to those obtained for “affect and motivation”.
Full Article Citation:
Brunner, M., Keller, U., Wenger, M., Fischbach, A., & Lüdtke, O. (2018). Between-School Variation in Students’ Achievement, Motivation, Affect, and Learning Strategies: Results from 81 Countries for Planning Group-Randomized Trials in Education. Journal of Research on Educational Effectiveness, 11(3), 452–478. https://doi.org/10.1080/19345747.2017.1375584.